学一门新的语言,第一个实战例子总是打印 hello world
;在机器学习领域,也有一个 hello world,那就是 MNIST
手写数字识别——当然,它可比打印字符串有意义多了。
什么是 MNIST
MNIST 是深度学习巨巨 Yann LeCun 维护的一套手写数字图像数据库,包含 60000 个训练样本和 10000 个测试样本,所有这些图像已经做过规范化及居中处理,拥有同样的固定尺寸,使用起来非常方便。下面是其中几个例子。
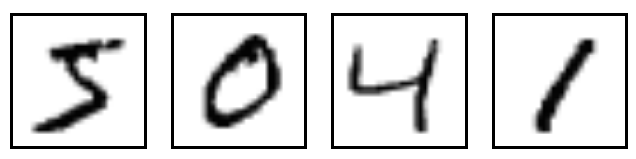
我们要做什么
很明显,我们马上要开始编写第一个 TensorFlow
程序,一个有实际应用价值的深度神经网络(DNN)
——虽然只有 2 个隐层,做 MNIST 手写数字识别,484 很鸡冻……
Let's do it, but how?
万事开头难,迈出第一步非常关键。为了避免陷入对无限发散的未知知识点(技能树)的恐慌,我们尽量将注意力集中在主线任务上,旁枝末节以后慢慢看,当然,楼主假设你还是会写基本的 Python
的。废话不多说,动手吧!
读取 MNIST 数据
from tensorflow.examples.tutorials.mnist import input_data
mnist = input_data.read_data_sets("MNIST_data/", one_hot=True)
input_data
封装了 MNIST 数据的下载、解析功能,read_data_sets()
返回三部分数据:55000 个训练样本 mnist.train
,5000 个验证样本 mnist.validation
(注意到没有,这 5000 是从原始的 60000 个训练样本上拿出来的),10000 个测试样本 mnist.test
。
每个样本都包含一个手写数字图像 x
和一个对应的标签 y
,训练集的图像和标签可以通过 mnist.train.images
和 mnist.train.labels
读取,验证集和测试集同理。图像的尺寸是 28x28 像素,每个像素值代表 [0,1] 的笔划强度,我们可以把图像数据理解为一个长度为 784 的数组,也就是一个 784 维的向量。标签的取值为 [0,9] ,表示从 0 到 9 这 10 个数字,这里我们把标签处理成 10 维 one-hot
向量以方便对应 DNN 的输出。
建立 DNN 模型
接下来我们搭建一个拥有 2 个隐层的 DNN
模型,第一层拥有 1024 个神经元,第二层拥有 625 个神经元,使用 ReLU
作为激活函数,并在输入层和隐层各层都使用 dropout
机制避免模型发生过拟合,这些概念暂时不懂也没有关系,把实验做完,不懂的再去查。
import tensorflow as tf
X = tf.placeholder(tf.float32, [None, 784])
Y = tf.placeholder(tf.float32, [None, 10])
p_keep_input = tf.placeholder(tf.float32)
p_keep_hidden = tf.placeholder(tf.float32)
def init_weights(shape):
return tf.Variable(tf.random_normal(shape, stddev=0.01))
w_h = init_weights([784, 1024])
w_h2 = init_weights([1024, 625])
w_o = init_weights([625, 10])
def model(X, w_h, w_h2, w_o, p_keep_input, p_keep_hidden):
X = tf.nn.dropout(X, p_keep_input)
h = tf.nn.relu(tf.matmul(X, w_h))
h = tf.nn.dropout(h, p_keep_hidden)
h2 = tf.nn.relu(tf.matmul(h, w_h2))
h2 = tf.nn.dropout(h2, p_keep_hidden)
return tf.matmul(h2, w_o)
py_x = model(X, w_h, w_h2, w_o, p_keep_input, p_keep_hidden)
训练模型
为了训练模型,必须有一个指标衡量模型的好坏,这个指标就是损失(loss 或 cost)
,loss 越接近于 0 表明模型的输出越接近于真实的标签,我们选择最常用的交叉熵 cross-entropy
作为损失函数, RMSProp
优化算法做梯度下降
。
cost = tf.reduce_mean(tf.nn.softmax_cross_entropy_with_logits(logits=py_x, labels=Y))
train_op = tf.train.RMSPropOptimizer(0.001, 0.9).minimize(cost)
评估模型
我们把模型输出的标签与真实的标签进行比较,并将比较结果转换为一个取值 [0, 1] 的浮点数作为准确率
指标。
predict_acc = tf.reduce_mean(tf.cast(tf.equal(tf.argmax(py_x, 1), tf.argmax(Y, 1)), tf.float32))
接下来,我们该启动程序了:
epoch_count = 20000
batch_size = 50
keep_input = 0.8
keep_hidden = 0.75
with tf.Session() as sess:
sess.run(tf.global_variables_initializer())
step = 0
for i in xrange(epoch_count):
step += 1
batch_x, batch_y = mnist.train.next_batch(batch_size)
sess.run(train_op, feed_dict={X: batch_x, Y: batch_y, p_keep_input: keep_input, p_keep_hidden: keep_hidden})
if step % 100 == 0:
loss, acc = sess.run([cost, predict_acc], feed_dict={X: batch_x, Y: batch_y, p_keep_input: 1., p_keep_hidden: 1.})
print("Epoch: {}".format(step), "\tLoss: {:.6f}".format(loss), "\tTraining Accuracy: {:.5f}".format(acc))
print("Testing Accuracy: {:0.5f}".format(sess.run(predict_acc, feed_dict={X: mnist.test.images, Y: mnist.test.labels, p_keep_input: 1., p_keep_hidden: 1.})))
整个训练过程共 20000 步,每步使用 50 个一组的随机样本做训练,每训练 100 步输出一次训练准确率,全部训练结束后使用测试集 mnist.test
评估准确率。
我们的 DNN 模型很简单,同时也很争气,准确率大概是 98.26%,有没有感觉到一种成就感……
小提示
虽然实验做完了,结果也还算不错,但此刻我们应该冷静下来认真做一下回顾,把整篇文章中不太明白的概念整理出来,深入地去做一下理论知识学习。这里推荐一本免费的电子书:Neural Networks and Deep Learning,拿来入门非常合适。